I spent the summer of 2022 visiting Markus Heinonen at Aalto University in Finland. Together we worked on energy conserving GP models, and I am happy to say our paper on the work was recently accepted to TMLR. Check out the paper, the code, or some visualizations.
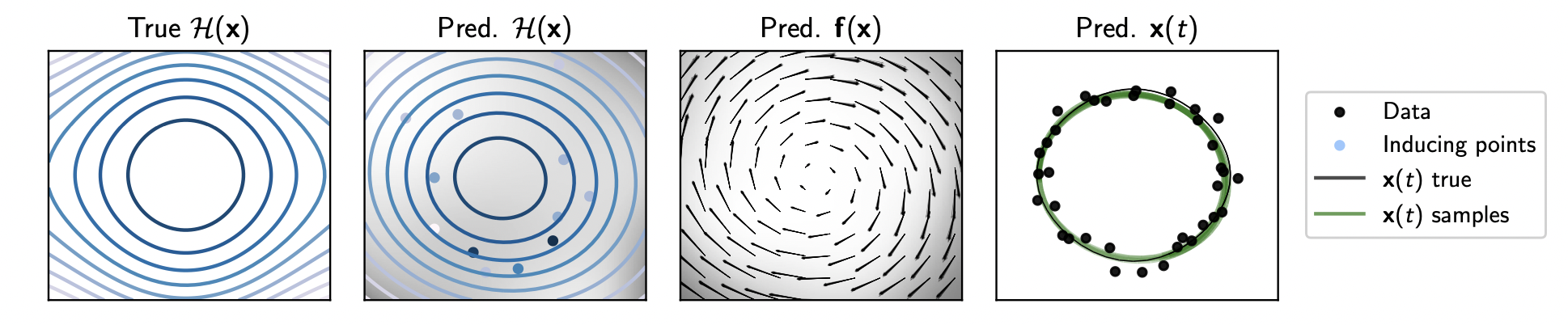
We place a GP prior over the Hamiltonian and, using a set of inducing points, map function samples through Hamilton’s equations to obtain system derivative samples, to which we apply ODE solver to obtain sample trajectories. Shading represents model uncertainty.